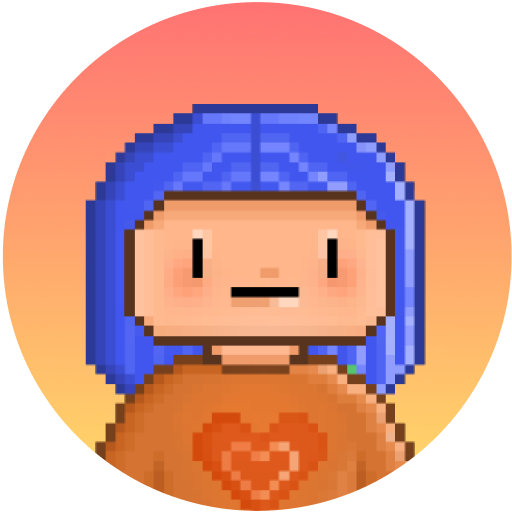
Garima Behal
September 22, 2023
•
20 min
Bring the fastest & most relevant semantic search to your software.
Try OSlash todayOffer 24*7 LLM-powered customer support to your users!
Try OSlash todayOffer 24*7 LLM-powered customer support to your users!
Try OSlash todayOffer 24*7 LLM-powered customer support to your users!
Try OSlash todayArtificial intelligence (AI) has moved beyond research labs to deliver real value across industries. As AI democratizes, anyone can now build tools that solve challenges big and small.
From virtual medical assistants that diagnose diseases to productivity copilots that take over our busy work, AI is lending humanity a helping hand everywhere imaginable.
But what are some of the most remarkable and empowering applications of AI?
This blog highlights innovative AI applications in domains such as healthcare, education, finance, and others. The examples demonstrate AI's vast potential to augment human capabilities when thoughtfully applied.
E-commerce has been one of the top adopters of AI, using it to optimize everything from shopping experiences to supply chains.
Here are the top five use-cases for AI for both e-commerce companies and their customers.
Using machine learning (ML) on behavioral data and content, recommendation engines filter content to anticipate consumer tastes and suggest relevant items. This helps contextualize marketing and increases both customer delight and company revenue. For instance, Amazon’s product recommendation engines power 35% of its revenue today.
But Amazon is not the only retailer making use of AI-powered search & recommendations.
China’s Taobao, owned by Alibaba, expands recommendations outside of products to live broadcasts, stores, brands, user-generated content (UGC) and professionally generated content (PGC), among other things. According to Alibaba, the basic components of the system include recommendation algorithms and models, raw logs and features obtained by processing log data, and offline computing and service capabilities, such as vector search, ML platforms, and online sorting.
{{cta-component-1}}
If you thought that the applications of AI in customer service were limited to chatbots and virtual assistants, think again.
Luxury department store Neiman Marcus uses visual AI to make it easier for customers to look for items. The ‘Snap. Find. Shop.’ app allows users to search through the Neiman Marcus inventory to find items based on pictures.
Starbucks’ AI-enabled voice ordering on their My Starbucks Barista app allows customers to order ahead and skip the line as they get to their local Starbucks.
The revolutionary Amazon GO stores make sure customers never have to go through a cashier to buy stuff they need. AI-powered cameras and sensors track in-store purchases, charging their Amazon accounts when they leave.
The bar for customer service in retail is HIGH thanks to AI!
Big retailers, whether online or offline, leverage AI to navigate demand-supply fluctuations and optimize inventories.
Walmart’s Intelligent Retail Lab (IRL) empowers employees with real-time inventory analysis. They no longer face the hassle of deciding which items need to be restocked when and in what quantity. The labs boast an “impressive array of sensors, cameras and processors connected by enough cabling to scale Mt. Everest five times and enough processing power to download three years’ worth of music (27,000 hours) each second”.
On a similar line, Swedish fast fashion brand H&M uses powerful ML algorithms to analyze store receipts, returns, and loyalty card data to better align supply and demand. In a pilot of the technology, their Stockholm store was able to reduce the number of items stocked by 40% based on intelligent inputs.
In India, the unicorn startup, Cars24, which operates in the used car retail and online marketplace sector, sets prices for its vehicles by using AI analysis of data collected from nearly half a million transactions. It also looks at data from over 300-point inspections performed on the cars, together with pictures and engine sounds to arrive at the right price in less than a second.
Fraud detection uses ML to identify suspicious activity from order, customer and payment patterns for e-commerce retailers. Once a company trains an AI model on that data, it can use it to look for pending transactions that are out of the ordinary, indicating potential fraud, and preventing the transactions from being completed.
A report by Juniper Research predicts that as fraudsters become more sophisticated, merchants will become more adept at using highly advanced AI-enabled fraud detection to combat crime. It adds that “cost savings from AI deployment will be critical to taking system use beyond regulatory compliance”.
Cost savings from AI fraud detection are expected to reach $10.4 billion globally in 2027, up from $2.7 billion in 2022.
In mid-2023, with the launch of Sidekick, Shopify's intelligent business assistant chatbot for entrepreneurs, AI assistants became the talk of the town. Sidekick is just one of the many capabilities on offer under Shopify Magic, Shopify’s catch-all Generative AI brand.
Powered by proprietary Shopify data in addition to available Large Language Models (LLMs), Shopify Magic can answer questions for business owners and merchants based on conversation histories and stories policies, help them summarize and strategize, assist in basic research, and even generate marketing content for them.
AI allows e-commerce and retail players to dynamically customize experiences, uncover insights, and optimize operations based on massive data. It has become a competitive necessity.
{{cta-component-2}}
AI is enhancing education through personalized and accessible learning for students and elimination of repetitive grunt work for teachers and educators.
According to Forbes, “Adjusting learning based on an individual student’s particular needs has been a priority for educators for years, but AI will allow a level of differentiation that’s impossible for teachers who have to manage 30 students in each class.”
This is exactly what Intelligent Tutoring Systems (ITS) like Carnegie Learning are aiming at. Cognitive models powering these software use learner profiles based on knowledge, engagement, and weak spots to target interventions. Students are provided with support when needed but also challenged to work independently.
Khanmigo, a GPT-4 powered learning assistant trained on Khan Academy’s vast library of educational content, is built on the same principles of personalized tutoring and feedback.
To use Khanmigo, students can ask questions about a topic they are learning, and Khanmigo will respond with hints, explanations, and examples. Khanmigo can also help students practice their skills by providing them with exercises and quizzes.
Smart content refers to educational materials that are enhanced and personalized through AI. These materials adapt to the needs and learning styles of individual students, providing a more engaging and effective learning experience.
A real-world example is the instructional design and content application solutions provided by Content Technologies Inc. Among other things, they empower instructors to create custom lecture series, syllabi, and textbooks. Their Cram101 technology is adept at turning any textbook into a smart study guide complete with summaries, tests, and flashcards.
For overburdened teachers, AI steps in to share the workload in the form of automated feedback and grading. Gradescope, for instance, helps teachers grade multiple students using AI-assisted grading and answer groups, reducing time spent on routine assignments.
Stanford recently trialed an AI system that critiqued thousands of student coding assignments in an online class. Neural networks trained on a decade’s worth of data from midterm test results power the system. This spring, the system provided 16,000 pieces of feedback, and students agreed with the feedback 97.9 percent of the time as compared to 96.7 percent of the time for human instructors.
Apps like Duolingo leverage natural language processing (NLP) to identify grammar and pronunciation mistakes and provide targeted feedback as students progress through the course.
Assistive AI technology, such as screen readers, speech-to-text tools, and alternative input devices, enables students with visual, hearing, mobility, or cognitive impairments to access materials and participate actively in learning activities.
For example, AI-powered language processing enables real-time transcription and captioning services for students with hearing impairments, enhancing accessibility in classrooms. Nuance, a speech recognition software, transcribes at an impressive rate of up to 160 words per minute. It is especially valuable for students who struggle with writing or have diverse accessibility needs.
AI also aids universities in plagiarism detection by analyzing vast amounts of text and comparing it against various online sources, academic databases, and previously submitted works. ML algorithms in tools such as Turnitin identify similarities, suspicious patterns, and potential instances of plagiarism, assisting institutions in maintaining academic integrity and ensuring originality in student submissions.
Overall, AI expands access to quality education while enhancing outcomes through data-driven personalization and automation.
AI and the Internet of Things (IoT) have led to the proliferation of smart wearables, medical monitoring devices, and fitness trackers that have made healthy living more accessible and cost-effective than ever before.
Moreover, during the COVID-19 pandemic, AI-based models emerged as powerful tools for predicting disease spread and optimizing resource allocation within healthcare systems.
Here’s how AI is helping doctors and health care professionals save lives.
AI is automating analysis and providing insights not possible by human doctors alone. Merative (formerly IBM Watson for Health) is helping healthcare organizations unlock vast amounts of health data from medical journals, symptoms, and case studies of treatment and response around the world.
Google’s DeepMind Health is working in partnership with clinicians, researchers and patients to solve real-world healthcare problems like giving doctors a 48-hour head start on life-threatening illness and avoidable patient harm.
On the other end of the spectrum, AI triage chatbots and virtual assistants like Babylon are providing medical guidance, book appointments, and process paperwork, allowing doctors to focus on the needs of patients.
PathAI is optimizing precision pathology with data from over 15 million annotations to “help accelerate drug development, improve confidence in the accuracy of diagnosis, and get life-saving therapies to patients more quickly”.
Moreover, wearable devices like the Apple Watch and Fitbit continuously track vital signs and can alert users to irregularities such as abnormal heart rhythms or sleep disorders. This real-time monitoring enables timely interventions and can potentially prevent serious health issues.
AI has significantly enhanced the efficiency and accuracy of medical imaging.
Google's DeepMind, for instance, has developed AI algorithms that can analyze retinal scans to detect diabetic retinopathy and other eye conditions. This technology is particularly promising for improving access to specialized medical expertise in remote areas.
Additionally, startups like Aidoc employ AI to assist radiologists in detecting abnormalities in CT scans, speeding up diagnoses and reducing the likelihood of human errors. Likewise, computer vision tools like Optellum detect cancerous lung nodules early through CT scans, with up to 10% better accuracy than radiologists.
AI has become a formidable force in accelerating drug discovery and development. Companies like Atomwise and Benevolent AI have emerged as trailblazers.
Atomwise employs deep learning to screen billions of chemical compounds, rapidly identifying potential drug candidates. Benevolent AI, on the other hand, leverages AI to analyze extensive datasets, uncovering novel drug targets and repurposing existing drugs for new therapeutic applications.
BPGbio is another example of a clinical stage biopharma that combines math, biology, and AI to accelerate and de-risk drug discovery.
These advancements hold the promise of expediting the delivery of life-saving medications to those in need.
ML is bolstering personalized medicine by predicting patient outcomes and helping determine the right interventions for each case. It improves recovery odds through data-based care.
Take Tempus as an example. They harness the power of AI to analyze both clinical and molecular data, enabling oncologists to tailor cancer treatments to individual patients. By considering genetic and clinical factors, AI assists in identifying the most effective and risk-free therapies.
Projects like InnerEye are helping analyze 3D body scans to plan precise radiation therapy personalized to a patient's anatomy, considerably reducing the side effects. It can segment images up to 13 times faster than doing it manually, “with an accuracy that is within the bounds of human expert variability”.
This not only leads to improved patient outcomes but also optimizes the allocation of healthcare resources.
AI-driven robots like the da Vinci Surgical System have ushered in a new era of precision in surgery. These robots offer surgeons enhanced dexterity and precision, resulting in higher success rates for minimally invasive procedures. For instance, in procedures like prostatectomies, the da Vinci system allows for smaller incisions, shorter recovery periods, and a reduced risk of complications.
Platforms like Johnson & Johnson's VELYS allow doctors to operate remotely with precision, expanding access to expert surgeons.
These advancements not only benefit patients by improving outcomes and access to care but also empower healthcare professionals with valuable tools and insights.
AI enhances agricultural efficiency, sustainability, and output in various ways.
AI-driven precision agriculture optimizes farming operations by collecting and analyzing data from various sources, such as satellites, drones, and sensors. This data helps farmers make informed decisions about planting, irrigation, and harvesting.
Weed detection tools spot infestations from drone imagery using object detection algorithms. John Deere's See & Spray technology, for instance, uses computer vision and ML to identify and selectively spray weeds, reducing herbicide use and improving crop yields.
AI models, computer vision systems and imaging technology, drones, and robots are being used to monitor the operational and financial health of farms, predict adverse weather patterns, and prepare for pest outbreaks. Climate Corporation's Climate FieldView uses AI to provide weather forecasts and pest risk assessments to farmers.
AI can also assist in estimating crop yields for resource allocation and market planning.
IBM's Watson Decision Platform for Agriculture utilizes an electronic field record (EFR) as a centralized and reliable source of information for each farm. The EFR is enriched with exclusive data sources. This includes highly accurate weather data from The Weather Company, soil information (moisture levels, nutrient content, fertility, and type), equipment data from IoT sensors, and farm practice data from cooperative growers (planting and harvesting dates, application rates of fertilizers and pesticides, harvest outputs). Additionally, high-definition visual imagery obtained from satellites and drones provides visual insights to support decision-making.
AI streamlines the agricultural supply chain by optimizing logistics, storage, and transportation. It ensures that crops reach markets or processing facilities efficiently, reducing waste.
AgriDigital is an example of a blockchain-based platform that uses AI to manage the supply chain, offering transparency and traceability for agricultural products.
As technology continues to advance, the integration of AI in agriculture will likely lead to increased yields, reduced resource consumption, and more resilient farming systems, addressing the challenges of feeding a growing global population while minimizing environmental impact.
AI is transforming finance through sophisticated predictions, personalization, and automation.
AI-driven software like Kensho and Quandl aggregate and analyze vast amounts of alternative data. Alternative data exists outside of traditional data sources such as company reports and can help investment professionals gain unique insights that inform their investment decisions.
Credit underwriting models also incorporate alternative data like education, social graphs etc. to complement traditional financial evaluation metrics and improve the quality of results.
Generative AI is a godsend for risk management because it can collate large amounts of information and help financial institutions analyze not just structured data (from reports, spreadsheets etc.) but also unstructured data (like bills & receipts, contracts, meeting transcripts etc.).
Forecasting models powered by ML can predict risks, lending rates, and market movements more accurately and quickly than linear models.
Financial institutions, therefore, employ AI models like IBM Algo Risk Service to analyze various risk factors in real-time, identify potential issues, and minimize losses.
Robo-advisory platforms like Wealthfront and Betterment leverage AI to create and manage automated and diversified investment portfolios for clients. These platforms use algorithms to tailor investment strategies to individual goals and risk tolerances, democratizing wealth management.
Personalized financial research assistants such as Quill, on the other hand, assist investors in searching through SEC filings and screening stocks on command, using natural language Q&A. In addition to summarizing key information for faster research, they also point investors right to the source, making it easy to audit the results.
AI-based software such as NICE Actimize is increasingly being used to ensure compliance with regulations and detect suspicious activities. These systems analyze transactional data and apply ML to identify potential money laundering, fraud, and regulatory compliance violations.
SteelEye, for example, provides solutions to “capture compliance data including orders, trades, communications, and market data” and uses AI to “unlock actionable insights while meeting compliance obligations for archiving, oversight, and reporting”.
Recently, PayPal Ventures also partnered with Deep Instinct, the first company to apply deep learning to cybersecurity, in order to help combat fraud and money laundering.
It’s evident that AI augments almost every financial activity with better predictions, efficiency, and insights.
The legal field is notorious for long working hours and burnout among lawyers. AI is automating legal drudgery while providing deeper insights to improve case outcomes.
The typical lawyer spends, on average, ~21% of their time conducting legal research, says ABA’s 2021 Legal Technology Survey Report.
AI-powered platforms like ROSS and LexisNexis Context use NLP to save lawyers time and money with relevant research served on a platter, almost instantly. They help lawyers avoid two dreaded situations:
Legal research platforms like Casetext's CARA suggest precedents and arguments by analyzing case law more deeply than keyword search. Improves win rates.
AI algorithms, like those employed by Relativity and Recommind, assist in the review of electronic documents during legal investigations and litigation. These tools can quickly flag relevant documents among massive datasets and potentially reduce the time and cost of legal proceedings.
AI-driven contract analysis software, such as Kira and ThoughtRiver, automates the review and extraction of key clauses and terms from contracts. This technology helps legal teams identify risks, obligations, and opportunities, making contract management more efficient.
AI-based predictive analytics tools, such as Lex Machina and Blue J Legal, reveal key insights on judges, lawyers, case data, and legal precedents to forecast the likely outcomes of legal disputes and inform case strategy. Attorneys and clients can make more informed decisions based on these predictions.
17% of people polled in the 2022 ABA Profile of the Legal Profession believe that AI tools will become mainstream in the legal profession in the next three years, from legal support chatbots on law firm websites to smart assistants who can free up lawyers from busy work.
{{cta-component-3}}
When we think about AI in transport and navigation, a few things come to mind.
Autonomous vehicles like Tesla’s. Travel time predictions and the fastest route suggestions from Google Maps. In-car personalization and infotainment based on driver profiles by BMW.
There are some even more spectacular yet unnoticed use cases and examples, though, which illustrate how AI is powering next-gen navigation and transportation solutions.
Through advanced traffic surveillance and control systems, AI helps manage traffic speed, detect lane departures, and exchange information with urban traffic control systems. Cooperative Intelligent Transport Systems (C-ITS) enable vehicles to communicate with each other and the infrastructure. This communication facilitates emergency braking warnings, distance sensing, collision avoidance, optimized intersection management, and more.
Deployed in 2016 by Alibaba Cloud in China, CityBrain is one such AI-powered traffic management platform that integrates data from various sources, such as surveillance cameras, GPS devices, and public transportation systems, to optimize traffic signal timings and manage traffic flow in cities. Post its implementation, the average traffic speed in the city of Hangzhou increased by 15%, and traffic violations were reported with 92% accuracy.
On Dubai roads, Intelligent Traffic Systems improved incident monitoring by 63% and emergency response by 30%. The Emirate has also developed 14 AI smart pedestrian crossing systems capable of detecting various road users, including vehicles, cyclists, and pedestrians, in order to enhance road safety.
Through Advanced Driver Assistance Systems (ADAS)—a combination of cameras, radars, and ML algorithms—AI provides a range of intelligent features that support drivers.
ADAS monitor blind spots, automatically brake in emergency situations, and assist in lane keeping, effectively reducing the risk of accidents and minimizing driver fatigue. For example, Tesla's Autopilot system utilizes AI to enable semi-autonomous driving, providing features like adaptive cruise control and automatic lane changing.
In addition to ADAS, there are driver monitoring systems that can detect driver drowsiness, distraction, and unsafe behavior, issuing alerts or taking corrective actions when necessary. Major automobile manufacturers like Mercedes-Benz and General Motors have begun implementing them to improve driver attentiveness and prevent accidents caused by driver impairment.
AI-driven logistics optimization plans vehicle routes and transportation based on demand forecasting and constraints.
Launched in 2019, Uber’s GENIE, “a novel architecture for deep learning creatively applied to temporal prediction, for instance, powered a 12.3% improvement in demand forecasting in over 100 cities worldwide”, helping the cab aggregator boost its operational efficiency.
Likewise, UPS uses big data and artificial intelligence (AI) to shave off millions of miles (and costs) from its delivery ops every year. It invests up to $1 billion in technology each year and has some noteworthy AI projects. The UPS Bot mimics human conversation and can answer frequent customer support queries related to package tracking, while ORION (On-road Integrated Optimization and Navigation), a proprietary tool that creates optimal routes for delivery drivers from customer, driver, and vehicle data, empowers UPS to reduce 100 million delivery miles annually!
AI-powered precise positioning combines data from cameras, lidar, and inertial sensors to pinpoint locations with high accuracy, down to a few centimeters. This capability not only improves traditional navigation but also enables augmented reality (AR) experiences.
An example is indoor navigation apps, such as Google's AR navigation, which can determine precise locations within buildings using visual landmarks, WiFi signatures, and sensors, letting users find their way more efficiently.
In the transportation sector, Uber’s AI-powered sensing and perception system leverages various sensors to overcome GPS limitations, enhance navigation accuracy, and detect potential crashes faster, improving safety and efficiency for both drivers and passengers.
AI augments almost every aspect of vehicles today—driving, maintenance, infotainment and more. It will be crucial for enabling fully autonomous transportation in the future.
AI is rapidly transforming the travel and tourism industry in innovative ways that enhance both the traveler experience and company operations.
Chatbots like those of Kayak, Expedia, and Hilton can field common customer inquiries, provide flight updates, modify reservations and more —streamlining communication and improving satisfaction.
Airfare prediction tools like Hopper leverage AI to analyze historical data and forecast future price changes, allowing travelers to book at opportune times and save money. Expedia notes their prediction tool has saved customers over $100 million on airfares.
Platforms like Booking.com and Tripadvisor employ sophisticated AI algorithms to study customer preferences and trip histories for crafting highly personalized trip itineraries and recommendations.
Other remarkable applications include:
Behind the scenes, AI drives revenue growth through dynamic pricing and inventory management. Airlines use AI systems like Amadeus Altéa to continuously adjust fares based on forecasted demand, maximizing revenue. Hotel platforms like Duetto optimize pricing and availability in real-time to capitalize on demand fluctuations.
Thanks to AR apps like Google Maps and AR City, you don’t need to be in a city to experience what it’s like.
Google’s Street View, for instance, transports you right to the precise location you select on Maps, enabling you to take a virtual tour from the comfort of your home.
These apps also provide travelers with interactive information about landmarks and points of interest, enhancing the accuracy and realism of these virtual experiences.
AI also breaks down language barriers for travelers through real-time translation apps like Google Translate and advancements in contextual multilingual chatbots. These innovations open up personalized and seamless global travel to millions of users worldwide.
In all these applications, AI promises more customized, streamlined, cost-effective and experiential travel—building traveler satisfaction and unlocking growth opportunities industry-wide.
Let users book trips and stays conversationally.
Explore OSlash Copilot for your app!
With the likes of autocomplete technology in IDEs like TabNine and A-Z copilots like the GitHub Copilot, AI is helping developers increase their coding speed 5x. Facebook’s Code Llama, an AI model built on top of Llama 2, is fine-tuned for generating and discussing code. It’s also free for research and commercial use.
Not just code generation, code reviews have also benefited from AI which can augment human reviews by analyzing quality, style, security etc and suggesting improvements.
Here are some of the ways AI is reshaping the world of software development, in addition to code automation and reviews.
LLMs like GPT-3 have unlocked powerful natural language capabilities for developers, but directly integrating them into apps remains challenging. Some companies are making LLMs more accessible to developers.
Hugging Face, for instance, is a popular platform and community that aims to democratize access to LLMs. It provides an extensive library of pre-trained models to developers through its Transformers library. This enables developers without deep learning expertise to implement powerful NLP features like sentiment analysis, text classification, language translation etc into their software without starting from scratch.
A number of businesses are solving for integrating specific AI capabilities into existing applications. OSlash offers companies out of the box semantic search and a copilot for their website, app, or software. Microsoft’s Copilot integrates AI into its Office suite of applications.
New LLM experiments such as the Gorilla LLM are taking LLM capabilities beyond language processing and providing ways for developers and researchers to effortlessly access and integrate various APIs using natural language, without the need for manual coding.
Ask any developer what they hate the most about their job and you’re likely to hear the word ‘documentation’. Documentation is as crucial for orgs as it is a hassle for developers.
AI-powered platforms, such as Mintlify and Docify, use NLP to assist software engineers in writing documentation and creating user-friendly experiences.
AI-enhanced CI/CD pipelines, such as Jenkins X and CircleCI, automate software deployment, monitoring, and rollback procedures. AI algorithms can optimize release schedules, reducing deployment failures and downtime.
AI-driven security tools, like Checkmarx and Veracode, identify vulnerabilities and potential security risks in code. These tools provide actionable insights to developers, enhancing software security.
AI empowers even non-coders to build apps of their own using low-code and no-code interfaces. Zapier allows automating workflows with minimal code using a visual interface, while Webflow is soon launching an AI component to speed up and simplify web development for non-experts.
As a result of all these innovative advancements, AI assists developers at every stage while expanding access to coding for everyone.
According to IBM’s Cost of a Data Breach Report 2023, organizations that use security AI and automation extensively save USD 1.76 million on average compared to organizations that don’t.
The use of AI overcomes the weakest link in the cybersecurity chain—human error. It safeguards data through advanced threat detection, accelerated response, and predictive modeling, as hackers and cyberattackers become infinitely smarter.
AI-driven security solutions like Darktrace and Blackberry’s Cylance utilize ML to identify patterns of suspicious behavior within an organization's network.
IBM’s Watson for Cyber Security also uses cognitive technology to analyze information—from countless blogs, articles, and threat intelligence reports to identify potential threats.
These systems can quickly and autonomously detect and respond to cyber threats, including malware and insider threats.
AI-powered behavioral analytics platforms, such as Splunk User Behavior Analytics (UBA) and Securonix, monitor user and entity behavior to detect anomalies. They help identify unusual patterns that could indicate a security breach or insider threat.
Similarly, Mastercard's Decision Intelligence solution applies ML to analyze and score real-time transactions, minimizing fraudulent transactions without hassling legitimate customers.
AI-enhanced email security solutions like Proofpoint and Barracuda Sentinel use advanced algorithms to detect phishing emails, malicious attachments, email compromise, and impersonation attempts.
AI-driven vulnerability assessment tools, such as Qualys and Tenable, scan IT environments automatically to identify vulnerabilities. ML on past scans and National Vulnerability Database (NVD) data help predict risks and prioritize patching efforts, focusing scarce security resources on critical issues.
A number of AI security tools also help simulate social engineering attacks, empowering security teams to spot potential vulnerabilities before cybercriminals can take advantage of them and infiltrate protected networks.
AI-powered security orchestration platforms, like Siemplify and Demisto, automate incident response workflows. They instantly execute playbooks to contain threats identified by ML with minimal human involvement.
In addition to these, there are security chatbots that handle threat alerts and suspicious activity reports to free up analyst time. Overall, AI augments human security teams with advanced automation, intelligence, and accessibility.
As we witness the exponential growth of computing power, it's clear that AI holds the potential to revolutionize every aspect of our lives.
Imagine living in smart cities where AI optimizes energy consumption, transportation, and public services. Picture self-driving cars effortlessly navigating our roads, reducing accidents and congestion. Envision robotics seamlessly integrating into businesses, streamlining operations and enhancing productivity. The possibilities are boundless.
However, it's crucial to remember that the true power of AI lies in its ability to augment human capabilities, not replace them. By thoughtfully developing AI systems that work alongside us, we can unlock a future filled with unprecedented levels of intelligence, personalization, and automation.